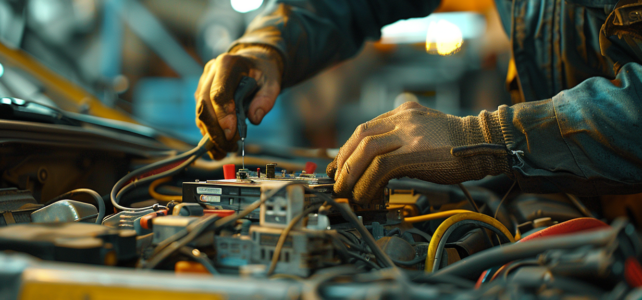
Au cœur des véhicules modernes, le calculateur joue un rôle fondamental, orchestrant la symphonie complexe des composants électroniques. Toutefois, il est parfois sujet à des défaillances, provoquant un spectre de problèmes mécaniques. Des erreurs de diagnostic aux capteurs défectueux, les…